In the seemingly never-ending quest to stay relevant and competitive in the tech industry the phrase “Jack of all trades, master of none” often rings true. Cybersecurity companies sometimes overengineer their offering as they try to cater to the needs of everyone in the market. This has been the case with data lakes offered for security purposes.
When Anton Chuvakin, aware of this trend, was reviewing his 2012 article on why DYI data lake projects will fail, he highlighted the high difficulty and costs of large-scale data analysis infrastructure and that traditional SIEMs are still hard to operate. Many organizations have turned to data lakes pushed by some newer versions of data lakes with reduced difficulty or costs of implementation.
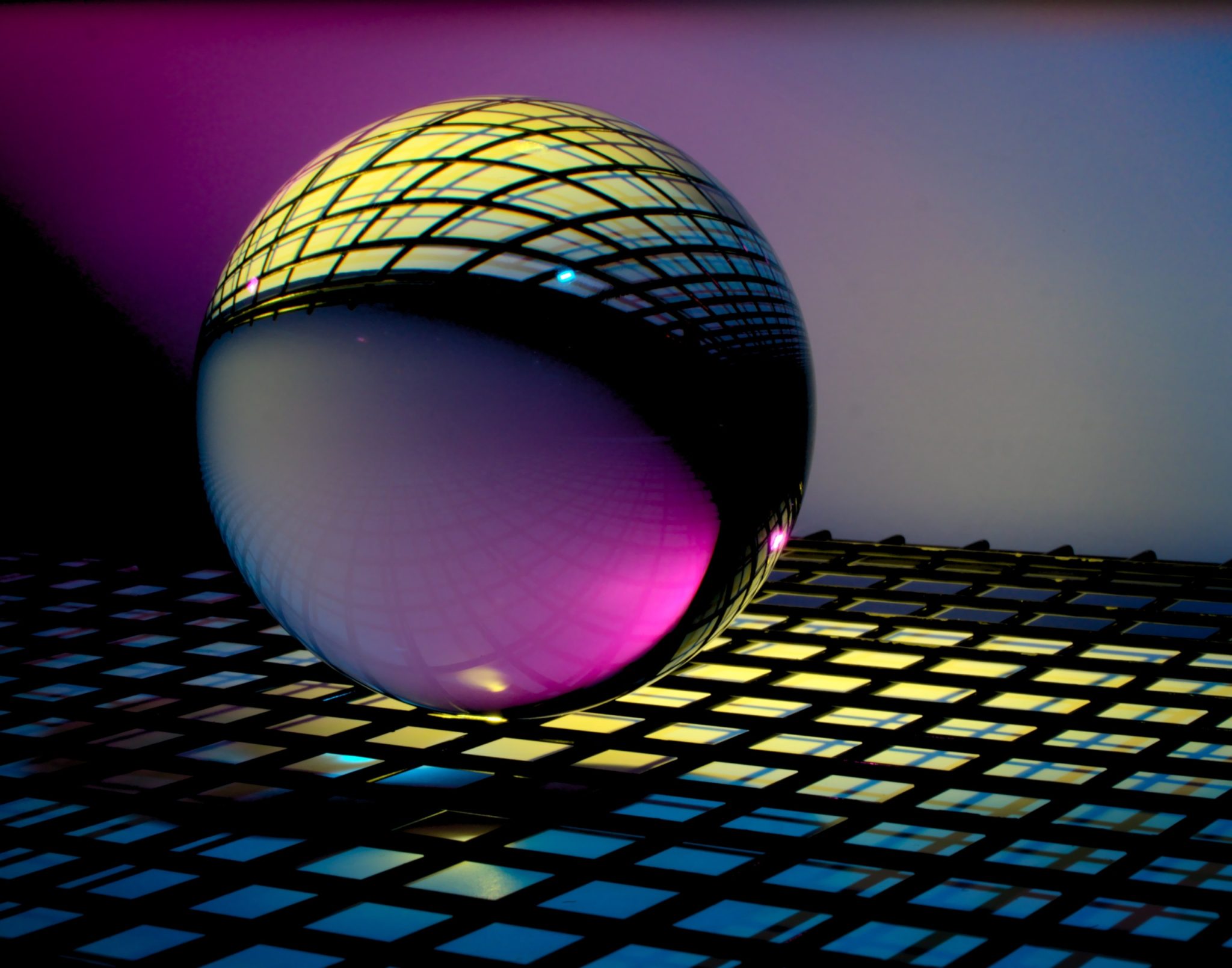
What Really is a Data Lake?
Organizations need more data sources as they grow and deal with different use cases. This has led to the proliferation of many log management and security solutions operating as “data lakes”.
A data lake by its common definition is for data storage in its native format and requires engineering-heavy teams to make sure the project succeeds. When discussing data lakes, it’s important to differentiate between data lakes in the real sense of the word and hybrid solutions, which are closer to how SIEM works than to data lakes. These SIEMs that sold themselves as hybrid solutions ingest data and index it over servers, instead of doing its native, raw format.
So, from this perspective, one could argue that SIEM solutions operate like security data lakes. And if companies start ingesting other security-related data that will contribute, such as IT Operations and Application Performance in the same platform, following this logic, the combination of these three into a single source of truth can hypothetically make up for a security data lake. Or perhaps not.
Multiple Sources of Truth vs. Data-Lake-Based Solutions
The rationale behind all this hoopla is that multiple sources of truth can cause friction between teams and having different solutions increases the costs. So, it’s best to have security, IT operations, and application performance data in one platform.
The argument of those in favor of this data-lake-based approach is that these solutions can provide tailored use cases by collecting and storing as much data as possible into a single platform.
While this can be true, such practice can also lead to the collection, storage, and processing of irrelevant data – not to mention the high costs of it. The reason for such a little return on investment is that the data sets required to address use cases in security, IT Operations and Application Performance will rarely overlap.
If the ingestion of all those events contributes poorly to the security altogether and the overlapping data is too narrow and will leave out a big chunk. Which do you ingest - everything, or the overlapping areas?
The Risks of Going for a Data-Lake-Based Approach
It’s obvious these are not data lakes in the true meaning of the word, but rather log management and SIEM solutions that aim to meet halfway the needs of data usage and optimization and they do that by removing the complex engineering a real data lake would require.
However, in the process of removing this complexity, they increase the need for more data structure and governance to ensure reliable collection. It’s not as simple as throwing data and fishing it out later. Otherwise, it’s likely that the data-lake project spirals out of control leaving them with a pool of unstructured data, also known as data swamp, and with high data collection costs.
In addition to the risk of organizations not knowing what they are doing, there’s also the risk of only focusing on the overlapping data sets required for use cases and leaving out the rest. However, if they wanted, this would be a costly task of nitpicking logs. Because even when the data source is employed for different use cases the logs can differ in each case.
Furthermore, only ingesting certain logs and ignoring others can lead to a lack of visibility, and attackers will always try to find the path of least resistance. So, in case of a data breach, analysts are blind to what happened in those areas.
This approach can make things more complicated in relation to requirements of data breach notifications, as happens with GDPR and NIS2. Then, organizations realize they are back to square one, and they must deal with ingesting all the data again.
The Logpoint Approach to Collecting Data
In conclusion, the problem with this lake-based approach in SIEM is that it’s prone to fail if companies don’t know how much data they need to ingest and what data they need for what use case. It’s not as simple as dumping all the data and fishing it later. Instead, they should have an unlimited budget if they’re willing to ingest all possible logs, as well as resources to build processes to avoid a data swamp.
On the contrary, Logpoint’s approach with Converged SIEM positions itself as a value-for-money solution. It comes with use cases and out-of-the-box configuration templates to provide companies with what they need. With licensing based on a number of nodes, or employees based on deployment, there’s no need to worry about increasing costs of data collection.
We understand that there’s no easy solution to friction between teams and organizations need to find the way that works best for them. Nonetheless, they must factor in the costs involved in these so-called data lakes and the risks if they are unsure about what they are doing. Or alternatively, they should opt for cost-effective SIEM solutions that can ingest a lot of data and store it at a low price but come with out-of-the-box use cases and customizable templates.