Data is at the center of every business model in our digitally interconnected world. We spend our days creating, using, and sharing data. All your employees use or have access to essential data, whether it’s a friendly message to a coworker or your clients’ private information.
Global cybercriminals and state-sponsored threat actors are constantly hunting for opportunities to steal, ransom, or sell our valuable data. To protect it, we need to understand how it’s handled. While traditional security tools can be effective against known threats, user and entity behavior analytics (UEBA) is exceptionally reliable for identifying unknown and internal threats.
UEBA is an innovative cybersecurity technology that uses machine learning algorithms to build a baseline of normal user behavior inside your network. In simpler terms, it takes note of everyone’s behavior.
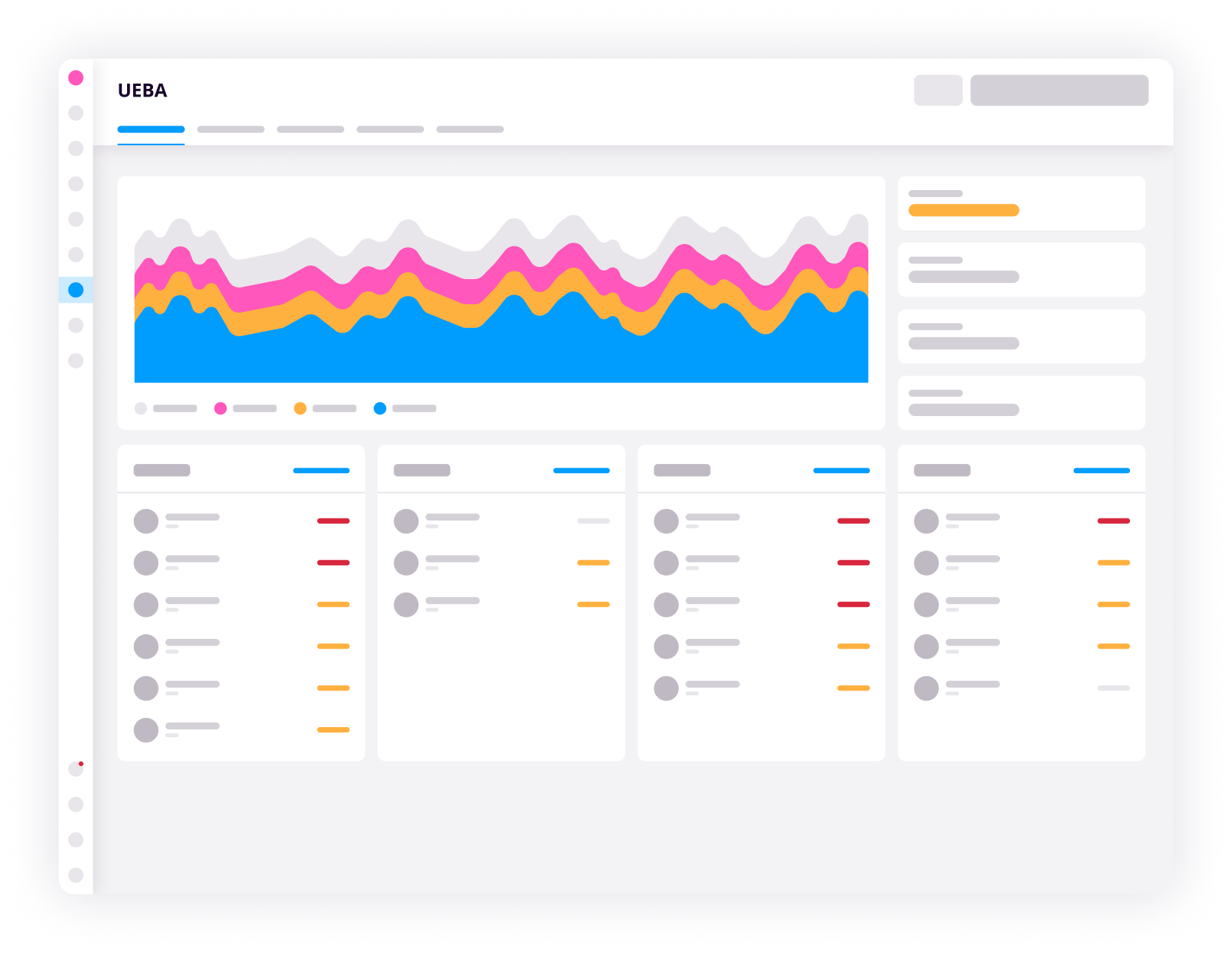
Once the algorithms learn normal behaviors, they can compare all-new actions with the expected normal behavior. Anything out of the ordinary immediately triggers an alert. As a result, you have an automated and more comprehensive way to detect anomalies that could put your entire infrastructure at risk.
To illustrate this, imagine one employee was attempting to access personal files with your clients' or customers' contact information. Still, they had never accessed it before and diverted from the expected behavior. UEBA identifies such events and correlates them to other suspicious events, enabling security teams to get a list of the riskiest entities and act on them before they cause damage. Detecting data breaches, policy violations, privilege abuse, and other insider threats far sooner and more efficiently helps limit the damage from attacks.
Cybercriminals constantly try to infiltrate your systems. And when they do gain access, it is often through employee error. According to Verizon’s 2021 Data Breach Investigations Report, ransomware's most common entry point is through phishing, representing 98% of incidents and 93% of breaches. Many breaches result from compromised user accounts because an employee was tricked into installing malware on their computer.
Once inside your system, criminals can change permissions and even create new users. In a worst-case scenario, they can access protected data, and your private information is no longer under your control.
Luckily, UEBA detects all these events. Even if adversaries target your cloud-based entities and third-party authentication systems, UEBA is designed to detect these attempts and allow analysts to block them.
The threat landscape is increasingly complex. It is now essential to use intelligent machine learning technologies, even for basic security processes.
Understanding history: UEBA vs. UBA?
UBA, or user behavior analytics, describes the process of tracking, collecting, and assessing user data and activities within the IT infrastructure.
In 2015, Gartner defined a more expanded term, UEBA, or user and entity behavior analytics. It offered the same capabilities as UBA and added the ability to track the activity of non-human entities, including devices, applications, and servers.
The fundamental difference is that UEBA combines user behavior with behavior from machines instead of just analyzing user behavior data. Every IT system is interconnected with humans and applications alike.
How UEBA uses machine learning
UEBA uses machine learning algorithms and statistical analyses to identify abnormal network activities. After UEBA builds a baseline for every entity's expected behaviors and actions in the network, it can then examine the data and evaluate all actions against these baselines.
With a stolen password, an adversary might break into a system. However, UEBA monitors all ongoing activities and catches subtle differences between behavior within your organization. An example of behavioral differences can be seen with peer grouping: UEBA doesn't just create a baseline for each user, but also teams or defined groups, etc. For example, if someone in a team accesses an unusual file for them, but the rest of their team accesses the file regularly, the behavior is not flagged and does not become a false positive because it's not abnormal for the team. UEBA monitors these subtle differences and creates a baseline comparison, reducing false positives when an individual does something new, which is otherwise a prevalent thing to do among the team. This dramatically increases your level of security.
As soon as the adversary logs in with a stolen password, UEBA will compare the details of what happens with the actual password owner's baseline of behaviors. To remain undetected, the hacker needs to successfully replicate another person's normal patterns. As soon as their actions don't make sense, UEBA points it out.
Because of its ability to process large amounts of data, machine learning is drastically more capable of identifying advanced threats than a human analyst. It can also identify and quantify behavioral patterns that a human analyst may not have considered.
The result is the detection of anomalies in all your systems, like applications, networks, file operations, and user actions. All abnormalities that break from the previous pattern get documented as potentially risky. The organization is then informed through automated alerts and the potential threats prioritized in importance, making it easy to manage them.
Machine learning can also recognize something unusual—even when the activity is not yet understood. By design, machine learning continues to 'learn' and conforms itself to the behaviors of legitimate users.
UEBA complementary to SIEM
Security information and event management (SIEM) is an essential technology that relies on rules to analyze data while providing real-time insight into data patterns and trends. Because of these rules, skilled adversaries can usually find a way to get around them.
And so, UEBA is a complementary tool to SIEM as it looks at employee behavior and is not rule-based. Instead, it uses advanced algorithms to detect risky anomalies that would be otherwise difficult to see in your SIEM.
The best practice is to use a mix of both. Your network is safer when you combine both UEBA and SIEM.
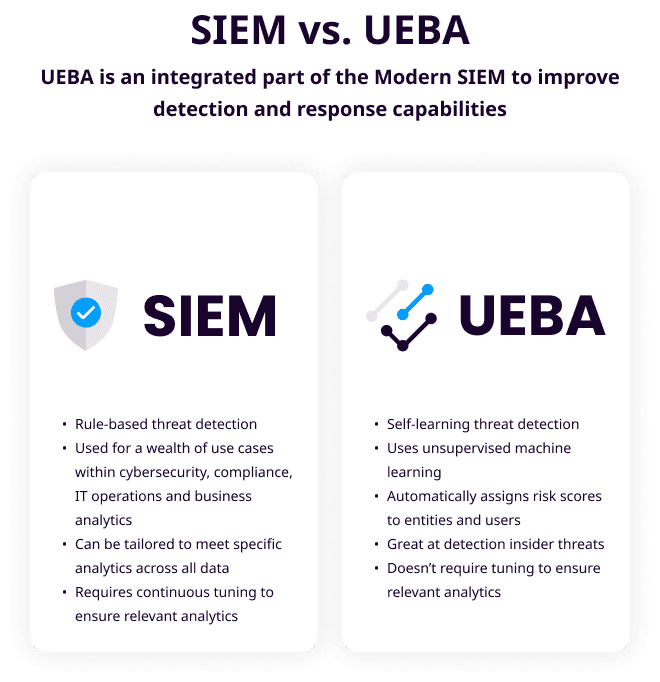
UEBA benefits organizations and security analysts
By integrating UEBA with SIEM, you can increase the number of security use cases you cover.
While UEBA provides insider threat detection, it can uncover both external attacks that have penetrated an organization’s perimeter and internal behaviors that may be threatening to company operations. UEBA also improves the effectiveness of existing security tools, supports entity monitoring, and helps organizations comply with industry regulations.
The behavioral analytics built into UEBA provides the answer to the question:
What is normal, and what is abnormal?
Without UEBA, analysts need to create complicated, predefined rules to define what is permitted. Since every individual in your organization has different habits, it would become a long list—especially if you employ hundreds of staff. And worse yet, it will never be definitive.
With UEBA, analysts gain the support of machine learning to track all users and entities and help determine what to look for. The powerful result is that UEBA provides analysts situational awareness before, during, and after responding to incidents.
Key benefits of UEBA:
- Automated threat detection: Using machine learning and behavioral analytics, enterprises can reduce the effect of the shortage of security analysts and optimize existing resources in threat detection. This includes detecting compromised accounts, brute force attacks, changes of permission, the creation of privileged users, and the breach of protected data.
- Reduced risk: Compromised user accounts provide cybercriminals with internal access to your network, resulting in loss or damage. Early detection of compromised credentials is essential in mitigating risk and data loss.
- Reduced mean time to respond (MMtR): UEBA uses high-fidelity risk scoring to reduce response time to attacks. The faster your security team is aware of an intrusion, the greater their ability to control it.
- Reduced noise: Behavioral analytics help eliminate false positives. In the context of increased threat loads, false positives can be overwhelming to a security team. Catching up on a backlog of alerts is an ongoing challenge for many security operations centers. With machine learning support, security teams have more time and ability to focus on uncovering activities providing the most significant risk and prioritizing responses to the most critical threats facing their organization.
Limitations of UEBA
Every cybersecurity tool has inherent strengths and limitations. And, unfortunately, adversaries know this as well. As such, criminals tend to focus their efforts on a particular software's limits to get past it.
To combat this, cybersecurity designers come up with new tools. That is why it is necessary to have an integrated platform of cyber tools. You get more comprehensive protection and insights when you have multiple strategies to protect your data. As threat actors become increasingly innovative and creative, protection technologies need to evolve to meet the challenge head-on.
Every business needs three essential requirements in its cybersecurity strategy to protect its digital assets and online systems. First, it's critical that you work from quality data. Without quality data—even the best machine learning algorithms are ineffective. The following requirement is integration. Communication between a SIEM and UEBA should be seamless for effective performance. Third, your security structure must be 'open' and capable of integrating advances in cybersecurity technology. Any UEBA lacking in these categories will deliver substandard performance.
While UEBA is good at identifying insider threats, it's also weak against the practice of concealing a message within a message, particularly images. As more hackers hide malware inside images, security measures require more specialized tools.
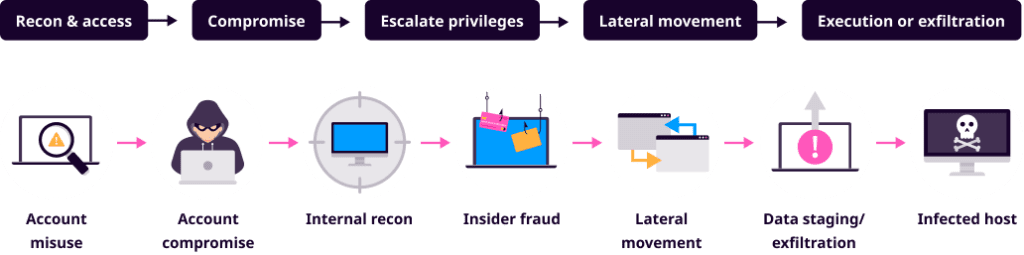
LogPoint SIEM integrates seamlessly with UEBA.
Many cybersecurity providers offer standalone cyber tools, but separate tools offer no overview and little ability to see patterns in the data. Beyond that, they require costly calibration and often time-consuming customization to work. Many also usually come with complicated structures and pricing structures that increase in proportion to data volume.
LogPoint SIEM is unique in the global cyber security landscape by successfully structuring data at ingestion into a common language. The high quality of data in LogPoint SIEM is essential for gaining value from machine learning and automation. As the data is in common language, it offers the ability to integrate in real-time with all LogPoint’s modular cyber tools – including UEBA.
LogPoint SIEM is an intuitive and cost-effective choice for analysts and organizations because of its out-of-the-box performance and precise ability to accelerate detection and response. Plus, LogPoint’s common language structure allows infinite future tool integration. As your protection needs evolve, so should your security systems.
LogPoint software's intelligent precision has helped hundreds of companies feel more confident in cybersecurity. LogPoint’s approach to scoring risky entities with a maximum out of 100 enables you to prioritize your time on the highest risks instead of other solutions with a constantly increasing score that makes it impossible to prioritize handling the highest risks. This advanced technology allows LogPoint to offer a commercial model with predictive pricing and no hidden costs. It's the perfect platform that scales with your business.
Contact LogPoint, and we'll help protect your company from cyber threats.
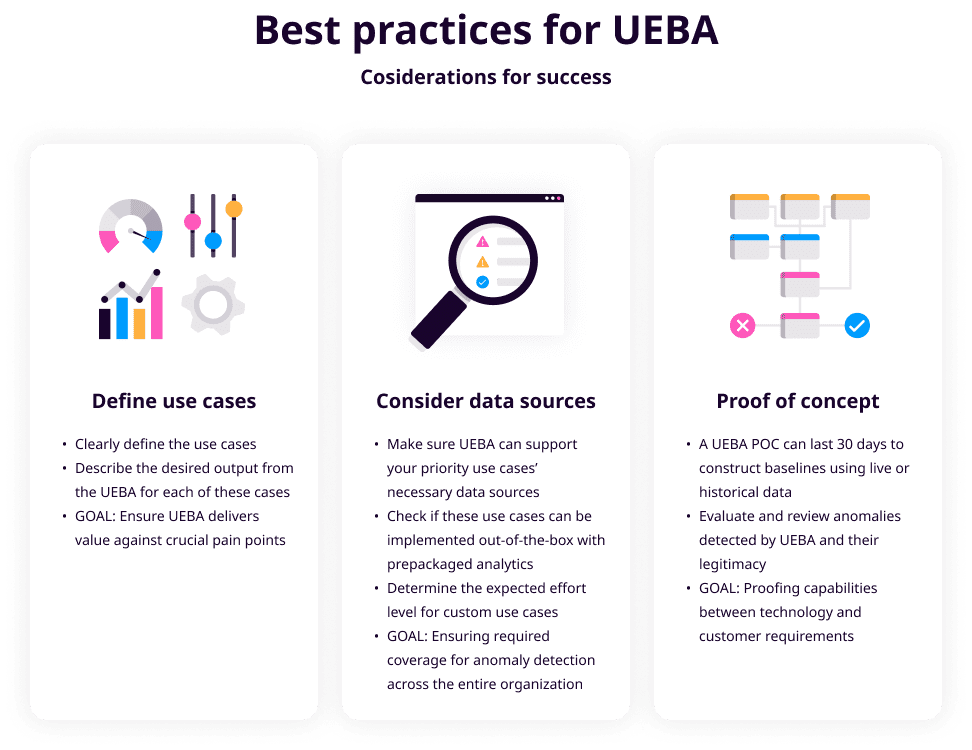